Artificial Intelligence for Drug Discovery
Our AI-enabled platform provides rigorously validated drug discovery strategies, precisely tailored to meet the unique challenges of each target.
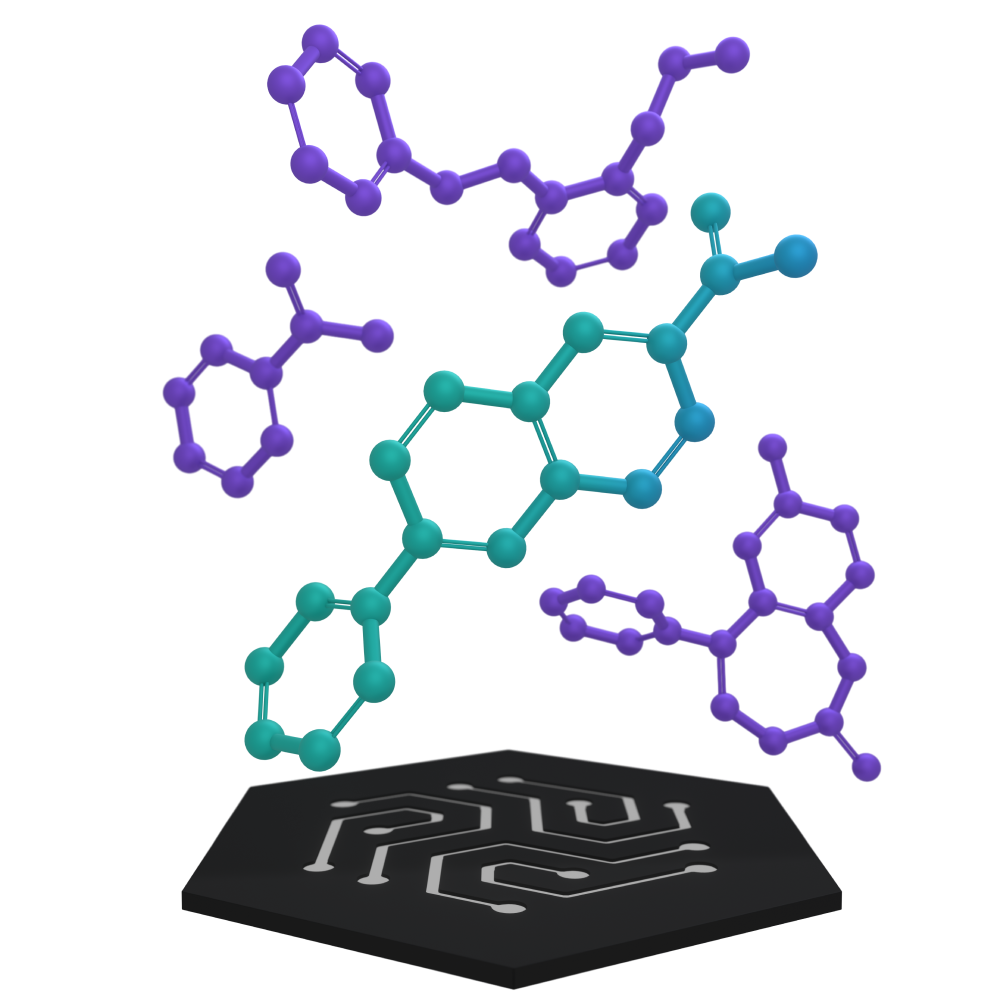
We employ a synergistic approach to hit identification by fusing advanced AI algorithms, physics-based computational methods, and real-time experimental feedback. This integrated methodology generates high-quality candidates that are primed for subsequent lead selection and optimization.

Generation of ultra-large chemical databases with specified properties.

Curated custom and focused chemical libraries (DNA-encoded, fragments, diversity, natural products, etc.).

Screening of large-scale multi billion chemical databases.

AI-assisted ligand- and structure-based virtual screening.

Molecular docking with custom-tuned AI scoring functions.

Assays for hit evaluation, in vitro screening and profiling.

Primary in silico and in vitro toxicity assessment.

Target molecule preparation (homology and ab initio modeling, structural quality assessment, binding sites identification).

Ultra-fast chemical space exploration (similarity searching, shape and electrostatic alignment and scoring).

Small molecules preparation and augmentation.

Drug-target interaction prediction using graph neural networks.

Rapid synthesis of designed hit compounds.

Primary in silico and in vitro target-specific selectivity assessment.

Optimized hit compounds are systematically transformed into a series of strategically designed derivative compounds. These are then evaluated for pharmacokinetic and toxicological profiles using an array of AI models, which are fine-tuned based on real-world experimental feedback.

Experimental feedback to improve bioactivity.

Structure-based optimization (using AI and SAR information).

ADME-Tox prediction (toxicity class identification, cytotoxicity, hepatotoxicity, acute toxicity, carcinogenicity, plasma protein binding, oral bioavailability, cytochromes inhibition, etc.).

Molecular dynamics and quantum calculations.

Molecular docking with custom-tuned AI scoring functions.

Experimental biochemistry and enzymology.

Exploration of the structure-activity relationships (SAR).

Off-target interactions evaluation using drug-target affinity predictive AI models.

Computer-aided drug design (in silico hit derivatives expansion and diversification, rapid synthesis and in vitro tests).

Advanced in silico and in vitro target-specific selectivity assessment.

Functional cellular assays.
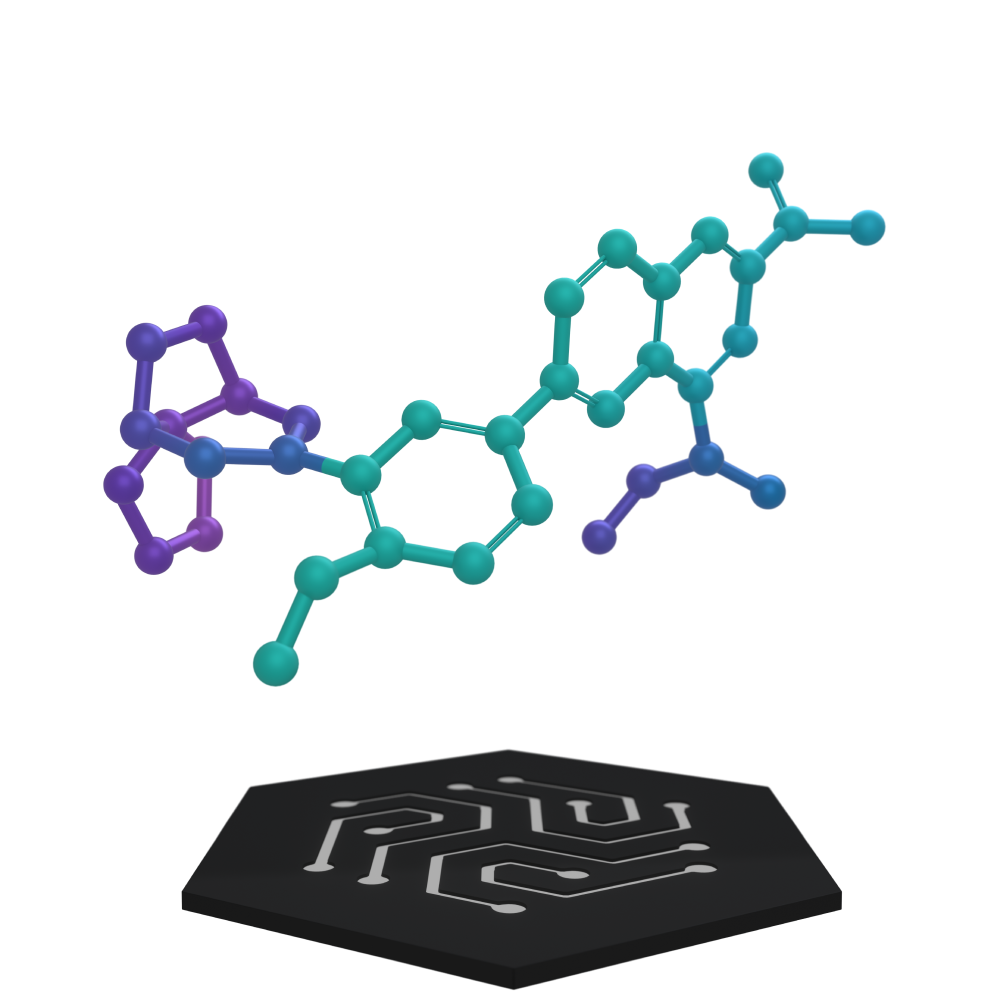
A powerful blend of AI algorithms and physics-based methods to fast-track your lead optimisations process. With machine learning for data-driven insights and molecular dynamics for structure refinement, we fine-tune lead compounds for optimal efficacy, safety, and pharmacokinetics.

Experimental feedback to improve safety.

Comprehensive polypharmacological profiles of the leads via knowledge graph.

Validation by molecular dynamics simulations and quantum chemistry techniques.

Ames test, Irwin's test, high-dose pharmacology, repeated dose toxicity in animal models, pharmacokinetics/ pharmacodynamics (PK/PD) studies, drug-induced metabolism exploration.

Confirmation of on-target and off-target effects.

Automated lead annotation.

Optimization of the structure-activity and structure-toxicity relationships.

Advanced medicinal chemistry and molecular optimization.

Solubility and permeability fine-tuning to increase bioavailability

Advanced ADME-Tox prediction (metabolism, biotransformation, biodistribution, compound excretion, Ames mutagenicity, etc.).

Comprehensive selectivity profiling with selected organ-specific targets.
Drug Candidate

Selectivity
Stability
Bioavailability